Inside Foundation Guardrails: A Technical Deep Dive into Our Enterprise-Ready AI Controls

As enterprises increasingly adopt AI into their systems, securing them and ensuring consistent performance in accordance with company policies and regulations becomes critical. AI guardrails play a key role here - these are specialized AI models that act as automated controls, monitoring and filtering AI system outputs. The DynamoGuard team understands this challenge deeply – that's why we offer Foundation Guardrails. We've built the expertise and technology stack needed to create reliable AI controls at scale, so your team doesn't need to build everything from scratch.
In this post, we'll pull back the curtain on how we build these guardrails, and why our approach delivers exceptional results for enterprises.
The Foundation Guardrail Advantage
Here's what makes them stand out:
Flexible Implementation:
Deploy our pre-validated Foundation Guardrails instantly, or tailor them to your exact needs. Unlike black box solutions, our guardrails let you refine both policy definitions and benchmark data.
Enterprise-Grade Performance
Foundation Guardrails are built for production environments, with high performance, low latency, and small size. These lightweight models easily embed into your AI applications with minimal latency increase.
Our guardrails achieve an average F11 of 0.94 on our human-curated benchmark datasets, withfalse positive rates under 10%. This ensures effective risk mitigation without reducing usability of your AI systems with a slew of false positives. Each guardrail undergoes comprehensive testing against adversarial attacks and edge cases, ensuring robust performance across diverse scenarios.
Built-in Policy Expertise
Our guardrails embed deep regulatory and compliance knowledge, particularly for financial services requirements. Each guardrail maps directly to specific regulations or guidance, like Article 5 of the EU AI Act, and includes implementation guidance based on real-world use cases. This integration of policy expertise means you're not just getting a technical solution – you're getting a ready-to-implement guardrail control that's informed by practical regulatory guidance and requirements.
Seamless Integration and Observability
Foundation Guardrails integrate easily with existing AI systems and provide comprehensive monitoring capabilities. This observability ensures you can track guardrail performance, maintain a log of flagged or blocked content, and quickly identify emerging issues to protect against.
From Implementation to Production
Foundation Guardrails provide four core components:
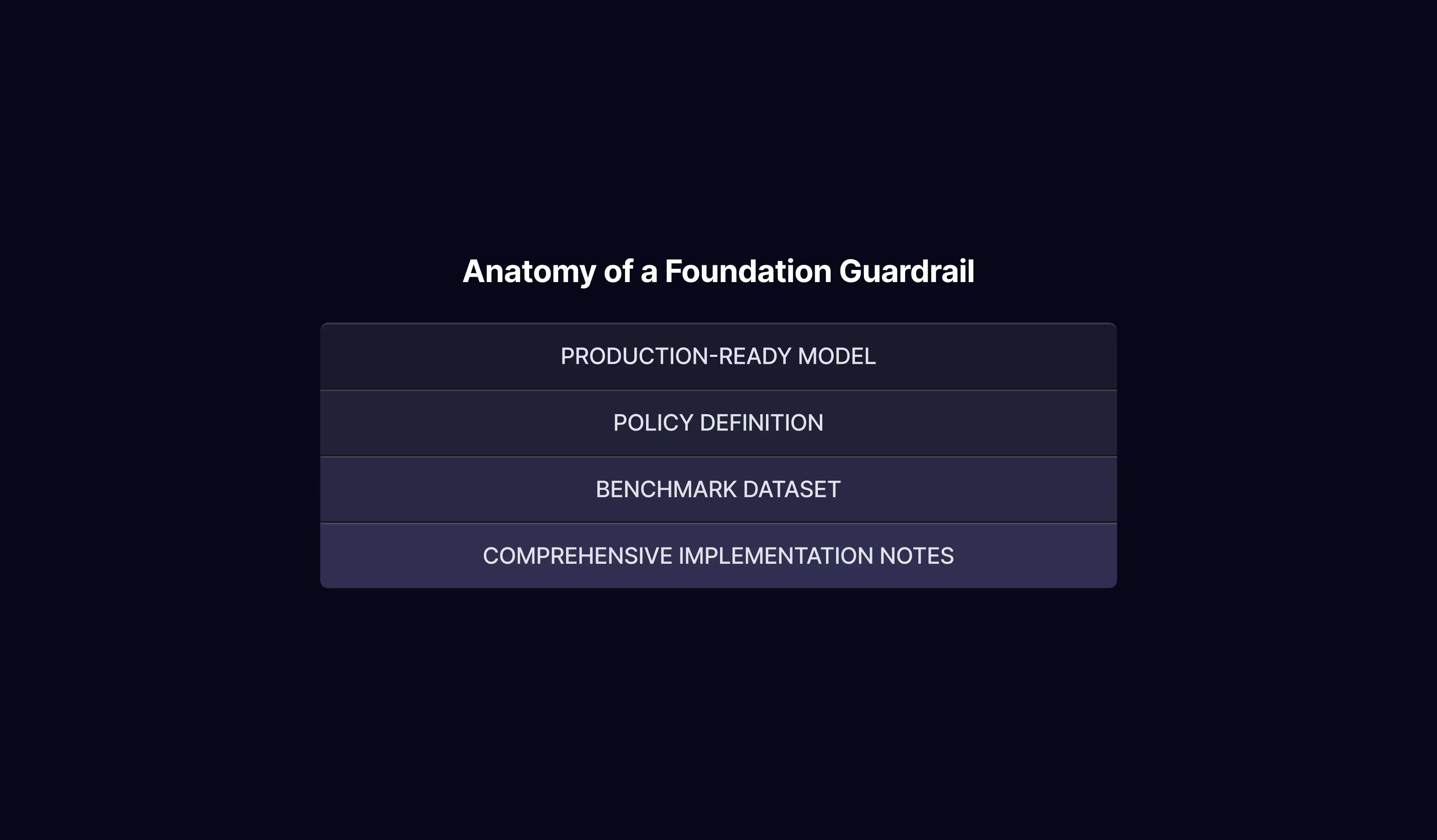
There are two paths to implementing Foundation Guardrails:

Behind the Scenes: Building Foundation Guardrails
In order to build reliable AI guardrails, we followed a comprehensive approach spanning policy research, specialized synthetic data generation, and rigorous redteaming. Here's a deep dive into our process.
Policy-First Development
We begin with intensive policy research, analyzing regulatory requirements and guidance, and mapping them to concrete implementation rules. This research phase involves decomposing complex policies into clear "allowed" and "disallowed" behaviors. This creates a foundation for an AI model to clearly understand the policy at its most granular levels.
Advanced Synthetic Data Pipeline
Training data quality determines guardrail performance. Our proprietary synthetic data generation methodology uses the policy definition and the application domain or use case to generate realistic and relevant compliant and noncompliant examples. The Dynamo AI Compliance Strategy and Product team reviews and provides feedback to our generation system. To ensure that our guardrail performs well across a variety of scenarios, we have our synthetic data pipeline generate different categories of training examples, such as:
- On-topic data representing expected use cases
- Adversarial examples that probe policy boundaries
- Borderline cases that test edge scenarios
- Jailbreaks that apply techniques to “trick” the model
- Diverse examples to enhance guardrail generalizability
This specialized approach ensures our guardrails can handle both straightforward cases and edge cases, is specialized for particular application domains, and is usable across many contexts.
Rigorous Redteaming and Validation
- Automated Evaluation with DynamoEval: Using DynamoEval tests, we subject guardrails to systematic perturbation testing, simulated jailbreak attempts, and edge case analysis.
- Manual Red Teaming: We conduct hands-on testing to test policy edge cases and challenge the boundaries of a policy.
We use insights derived from this testing to improve the guardrail. Finally, we only release a guardrail if it achieves at least 0.9% F1 score on our benchmark dataset, with a sub-10% false positive rate.
1An F1 score is a measure of accuracy that combines precision (avoiding false alarms) and recall (catching true cases). It ranges from 0 to 1, with 1 being perfect performance.
Ready to protect your AI systems with production-grade guardrails? Contact us to see our Foundation Guardrails in action.