DynamoGuard’s Reinforcement Learning from Human Feedback (RLHF) Guardrail Improvement

Limitations of Static Guardrails
AI guardrails are critical to successfully deploying generative AI in production, mitigating risks like prompt injection attacks, model misuse, or non-compliant model responses. Without robust guardrails, enterprises face significant challenges in ensuring reliable AI performance. However, today’s out-of-box guardrail solutions often fall short in real-world scenarios.
Real-world AI usage differs significantly from benchmarking datasets. It’s nuanced, diverse, and constantly evolving. At the same time, new prompt injection and jailbreaking attacks constantly emerge, making it challenging for static guardrails to keep pace with the latest vulnerabilities. As a result, out-of-box guardrails can fail to effectively safeguard these complex, evolving production environments, leading to issues such as
- False Negatives: Guardrails fail to catch unsafe or non-compliant content. This can be especially problematic as new vulnerabilities and prompt injection attacks constantly emerge - static guardrails are not adapted to address these.
- False Positives: Guardrails incorrectly detect legitimate content as being unsafe or non-compliant, hindering user experiences and limiting model utility
Recognizing these challenges, we’re excited to announce DynamoGuard’s RLHF (Reinforcement Learning from Human Feedback) Guardrail Improvement feature. This new capability empowers enterprises to dynamically adapt guardrails based on real-world production data, enhancing reliability and safety.

Deep Dive: DynamoGuard’s RLHF Guardrail Improvement
RLHF Guardrail Improvement enables technical and non-technical users to iteratively improve guardrail performance in production scenarios, using the following steps:

- Real-Time Monitoring: DynamoGuard automatically captures monitoring logs with user inputs, model responses, and guardrail outputs from production scenarios.
- Leave Feedback on Monitoring Logs: Users can review monitoring logs and directly provide feedback in the DynamoGuard platform. For example, users can annotate logs to be false positives or false negatives.
- Apply Feedback to Policies: Logs with feedback are sent to guardrails, where users responsible for maintaining the policy can choose to apply the feedback for guardrail improvements.
- Update Guardrail Models: After being applied, the feedback is used to retrain the guardrail model. Under the hood, this adjusts the guardrail’s understanding of the guardrail policy and improves its ability to distinguish between compliant and non-compliant examples in real-world contexts.
By continuously going through this process, enterprises can ensure that their guardrails remain effective, even as usage patterns evolve and new vulnerabilities emerge,
Real-World Results: Case Study
To demonstrate the impact of the RLHF Guardrail Improvement feature, we worked with enterprise customers in the finance sector to adapt a policy designed to prohibit personalized financial advice.
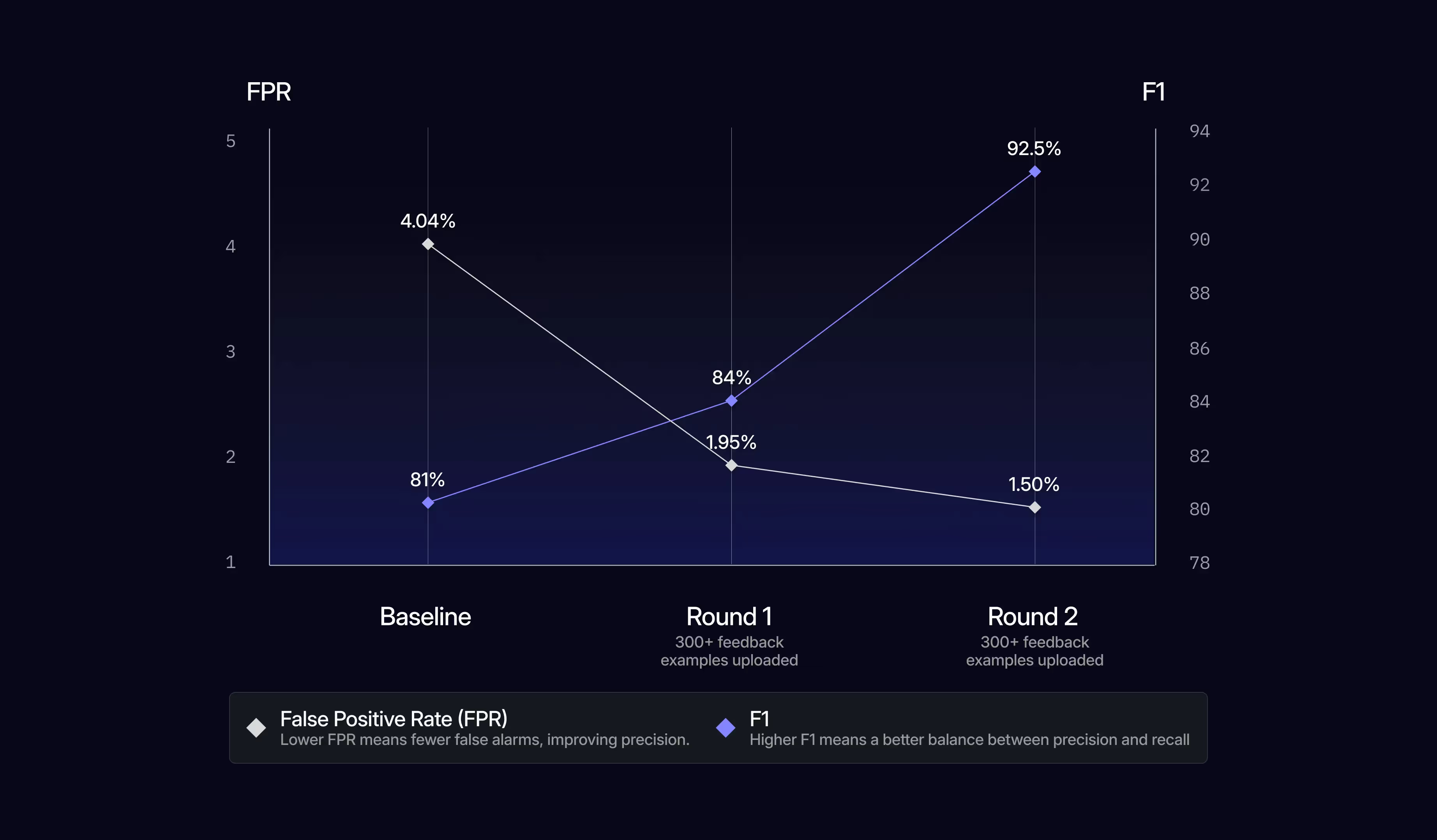
When first deployed, a set of 6 guardrail models achieved a collective 81% F1 and 4.04% FPR on a real-world sample set. While these results were promising, customer feedback highlighted several challenges:
- The guardrails flagged legitimate user requests for general financial information as non-compliant, preventing users from getting essential information, such as details about the company’s consumer security protections
- Certain non-compliant examples bypassed the guardrails, such as gibberish requests or attempts to disclose system prompts
Iterative Feedback Process
Round 1
300+ feedback examples were uploaded by the customer, spanning the 6 policies. After retraining the guardrail with this feedback, the policy improved to 84% F1 and reduced the FP rate to 1.95% on the real-world samples..
Round 2
Another 250+ feedback examples were uploaded to DynamoGuard. After retraining the guardrail with this feedback, the policy improved to 92.5% F1 and 1.50% FP rate on the real-world test data.
Final Outcome
Through just these rounds of feedback, the policy demonstrated measurable improvement in handling nuanced, real-world scenarios. This iterative process showcased how RLHF Guardrail Improvement adapts dynamically to evolving usage patterns, ensuring robust and reliable compliance.
Get Started Today
DynamoGuard’s RLHF Guardrail Improvement feature represents a significant advancement in deploying generative AI safely and effectively by ensuring that guardrails are not static, but instead adapt and grow smarter over time. By leveraging real-world data and human insight, enterprises can enhance safety, improve AI system utility, and build trust through transparent compliance practices.
Book a demo today!